The fusion of quantum computing and machine learning has emerged as a beacon of innovation, paving the way for unprecedented advancements. At the forefront of this revolutionary convergence lies the captivating field of Quantum Machine Learning (QML). This article delves into the depths of QML, unraveling its intricacies and exploring the profound impact it holds for the future of computing and artificial intelligence.
Definition of Quantum Machine Learning
Quantum Machine Learning, often abbreviated as QML, represents the amalgamation of quantum computing principles with the powerful algorithms of machine learning. To comprehend the significance of this union, it's essential to grasp the fundamental concepts that define both quantum computing and machine learning.
Quantum computing harnesses the principles of quantum mechanics, a branch of physics that delves into the behavior of matter and energy at the most fundamental level. Unlike classical computing, which uses bits to represent information as either 0s or 1s, quantum computing leverages quantum bits or qubits. These qubits can exist in multiple states simultaneously, thanks to the phenomenon of superposition, enabling quantum computers to process vast amounts of data in parallel.
On the other hand, machine learning empowers computers to learn and make decisions without explicit programming. It relies on sophisticated algorithms that enable systems to recognize patterns, make predictions, and continuously improve through experience. The synergy of quantum computing and machine learning opens new frontiers by enhancing computation speed and processing capabilities, promising breakthroughs in solving complex problems that were once deemed insurmountable.
As we embark on this exploration of Quantum Machine Learning, we will unravel the intertwined threads of quantum mechanics and machine learning algorithms, delving into the realms of superposition, entanglement, and the promising applications that await in the quantum landscape. Join us on this enlightening journey into the heart of a technological revolution that holds the potential to redefine the boundaries of what machines can achieve.
Table of Contents
I. Understanding Quantum Computing
To truly appreciate the groundbreaking synergy of Quantum Machine Learning (QML), it's crucial to establish a foundational understanding of the underlying principles of quantum computing. This section takes you on a journey through the fascinating world of quantum mechanics and the innovative landscape of quantum computing.
Basics of Quantum Mechanics
At its core, quantum mechanics is a branch of physics that explores the behavior of matter and energy on the smallest scales, where classical physics falls short. Quantum mechanics introduces us to a realm where particles, such as electrons and photons, exhibit behaviors that defy our classical intuitions. Two fundamental principles, superposition and entanglement, lie at the heart of quantum mechanics.
Superposition: In the quantum world, particles can exist in multiple states simultaneously. Unlike classical bits that can be either 0 or 1, quantum bits or qubits can be in a superposition of both states. This unique property enables quantum computers to perform parallel computations, exponentially increasing their processing power.
Entanglement: Entanglement is a phenomenon where two or more particles become interconnected, and the state of one particle instantaneously influences the state of the others, regardless of the distance between them. This interconnectedness is a key feature leveraged in quantum computing to facilitate complex computations.
Quantum Computing Principles and Operations
Quantum computing harnesses the principles of quantum mechanics to process information in ways that classical computers cannot. Quantum bits, or qubits, represent the basic units of quantum information. Unlike classical bits, qubits can exist in a superposition of states, exponentially expanding the computational possibilities.
Quantum gates are the building blocks of quantum circuits, manipulating qubits to perform computations. The intricate dance of quantum gates, guided by principles of superposition and entanglement, allows quantum computers to solve problems that would be impractical for classical computers.
As we navigate the intricacies of quantum computing, it becomes evident that this paradigm shift holds profound implications for the field of machine learning. The ability of quantum computers to process vast amounts of data in parallel opens new avenues for developing powerful machine-learning algorithms, propelling us into an era where computation transcends the limits of classical paradigms.
II. Foundations of Machine Learning
With a solid grasp of quantum computing principles, we now turn our attention to the bedrock of machine learning—the set of algorithms and concepts that have fueled transformative advancements in artificial intelligence.
Core Concepts in ML
Machine learning operates on the premise of enabling computers to learn from data and improve their performance over time without being explicitly programmed. The core concepts of machine learning lay the groundwork for this dynamic and evolving field.
Supervised Learning: In supervised learning, algorithms are trained on a labeled dataset, where each input is associated with a corresponding output. The algorithm learns to map inputs to outputs, making predictions on new, unseen data.
Unsupervised Learning: Unsupervised learning involves working with unlabeled data. The algorithm explores the inherent structure of the data, identifying patterns and relationships without predefined categories.
Reinforcement Learning: Inspired by behavioral psychology, reinforcement learning involves an agent interacting with an environment and learning to make decisions by receiving rewards or penalties. It excels in scenarios where an algorithm must make a sequence of decisions to achieve a goal.
Key Algorithms and Techniques
Machine learning algorithms are the driving force behind the ability of systems to learn and adapt. Understanding these algorithms is crucial for unlocking the potential of Quantum Machine Learning.
Linear Regression: A fundamental algorithm for predicting a continuous variable based on input features. It establishes a linear relationship between the input variables and the target variable.
Decision Trees: Decision trees are hierarchical structures that make decisions based on the values of input features. They are used for both classification and regression tasks.
Neural Networks: Inspired by the structure of the human brain, neural networks consist of interconnected layers of nodes (neurons). Deep learning, a subset of machine learning, leverages neural networks to model complex patterns.
III. Merging Quantum and Machine Learning
The fusion of Quantum Computing and Machine Learning heralds a transformative era in the landscape of technological innovation. In this section, we explore the seamless integration of quantum and machine learning, unraveling the advantages and challenges that arise in this groundbreaking union.
Advantages and Challenges
1. Advantages of Quantum Machine Learning (QML)
Exponential Processing Power: Quantum computers, leveraging the principles of superposition and entanglement, can process vast amounts of data in parallel. This exponential increase in processing power holds the potential to solve complex problems at speeds unattainable by classical computers.
Enhanced Optimization: Quantum algorithms excel in optimization tasks, offering efficient solutions for combinatorial optimization problems that permeate various industries, from logistics to finance.
Parallelized Training of Models: QML allows for the parallelized training of machine learning models, significantly reducing the time required to train complex algorithms.
2. Challenges in Merging Quantum and Machine Learning
Quantum Decoherence: Quantum states are fragile and susceptible to environmental interference, leading to a phenomenon known as decoherence. Maintaining quantum coherence over extended periods poses a significant challenge in practical implementations.
Error Rates and Quantum Noise: Quantum computers are prone to errors due to factors such as quantum noise and imperfections in hardware. Addressing these errors is crucial for ensuring the reliability of quantum machine learning algorithms.
Resource Intensiveness: Building and maintaining quantum computers requires specialized and resource-intensive environments. Overcoming the resource challenges associated with quantum computing is a key hurdle in widespread adoption.
Quantum Machine Learning Algorithms
Quantum machine learning algorithms leverage the unique properties of quantum computing to enhance classical machine learning tasks.
Quantum Support Vector Machines (QSVM): QSVM offers a quantum speedup for classification tasks, demonstrating advantages over classical support vector machines.
Quantum Neural Networks: Quantum neural networks harness the power of quantum computing to model complex patterns, presenting an innovative approach to deep learning.
Quantum k-Means Clustering: This quantum algorithm provides an efficient solution for clustering tasks, a fundamental aspect of unsupervised learning.
IV. Applications and Use Cases
Quantum Machine Learning (QML) opens doors to a multitude of applications and use cases, redefining possibilities across various industries. In this section, we delve into the tangible impact of QML, exploring the realms where its transformative potential is coming to fruition.
Industries Benefitting from QML
Healthcare and Drug Discovery: Quantum Machine Learning accelerates the process of drug discovery by optimizing molecular simulations and predicting molecular interactions. This has the potential to revolutionize healthcare by expediting the development of new medicines and treatments.
Finance and Optimization: In the financial sector, QML is leveraged for portfolio optimization, risk assessment, and fraud detection. The ability to process vast datasets in parallel enhances the accuracy and efficiency of financial models.
Logistics and Supply Chain: QML algorithms contribute to optimizing logistics and supply chain management. From route optimization to inventory management, QML provides solutions to complex combinatorial optimization problems.
Climate Modeling and Environmental Impact Assessment: Quantum computing, coupled with machine learning, facilitates advanced climate modeling and environmental impact assessments. This enables more accurate predictions and informed decision-making to address global challenges.
Real-world Implementations and Success Stories
Google's Quantum AI Lab: Google has been at the forefront of quantum computing research, with its Quantum AI Lab exploring applications such as optimization, cryptography, and machine learning. Google's quantum supremacy experiment demonstrated the potential of quantum computers to outperform classical computers in specific tasks.
IBM Quantum for Business: IBM offers quantum computing services for businesses, including the development of quantum machine learning algorithms. Industries such as finance and energy are leveraging IBM's quantum capabilities to solve complex problems.
D-Wave's Quantum Annealing: D-Wave Systems specializes in quantum annealing, a type of quantum computing. Their systems have been applied to optimization problems in fields such as finance, healthcare, and logistics.
Rigetti Computing's Hybrid Quantum-Classical Systems: Rigetti Computing focuses on hybrid quantum-classical systems, combining the strengths of both classical and quantum computing. This approach shows promise in various applications, including machine learning tasks.
V. Future Possibilities and Innovations
As we stand at the intersection of Quantum Machine Learning (QML), the horizon unfolds with promising possibilities and transformative innovations. In this section, we peer into the crystal ball, exploring the potential transformations in technology that QML may usher in.
Potential Transformations in Technology
Supercharged Artificial Intelligence (AI): Quantum Machine Learning has the potential to supercharge AI capabilities. The ability to process complex datasets at unprecedented speeds could lead to more advanced natural language processing, image recognition, and decision-making systems.
Secure Communication: Quantum computing's unique properties, such as quantum key distribution, could revolutionize cybersecurity. Quantum-secure communication methods promise unbreakable encryption, ensuring the privacy and integrity of sensitive information.
Optimized Machine Learning Models: Quantum computing's optimization capabilities could enhance the training and optimization of machine learning models. This could lead to more efficient and accurate models across various domains, from healthcare diagnostics to financial predictions.
Simulating Quantum Systems: Quantum computers are inherently well-suited for simulating quantum systems, a task that classical computers struggle with. This capability could revolutionize our understanding of complex quantum phenomena and drive advancements in materials science and chemistry.
Predictions and Developments in Quantum Machine Learning
Widespread Adoption: As quantum computing technologies mature and become more accessible, the adoption of Quantum Machine Learning is likely to increase across industries. Businesses and researchers may harness the power of quantum computing to solve previously insurmountable problems.
Hybrid Quantum-Classical Systems: The development of hybrid quantum-classical systems, where quantum and classical processors work in tandem, is expected to play a pivotal role. This approach combines the strengths of both paradigms, addressing the challenges posed by quantum decoherence and error rates.
Quantum Machine Learning as a Service (QMLaaS): With the evolution of cloud-based quantum computing services, Quantum Machine Learning as a Service may become a reality. This would enable businesses to access quantum computing power without the need for substantial infrastructure investments.
Interdisciplinary Collaboration: The interdisciplinary nature of Quantum Machine Learning is likely to foster collaboration between quantum physicists, computer scientists, and domain experts. This collaborative approach could lead to groundbreaking discoveries and innovations.
VI. Recommend Book
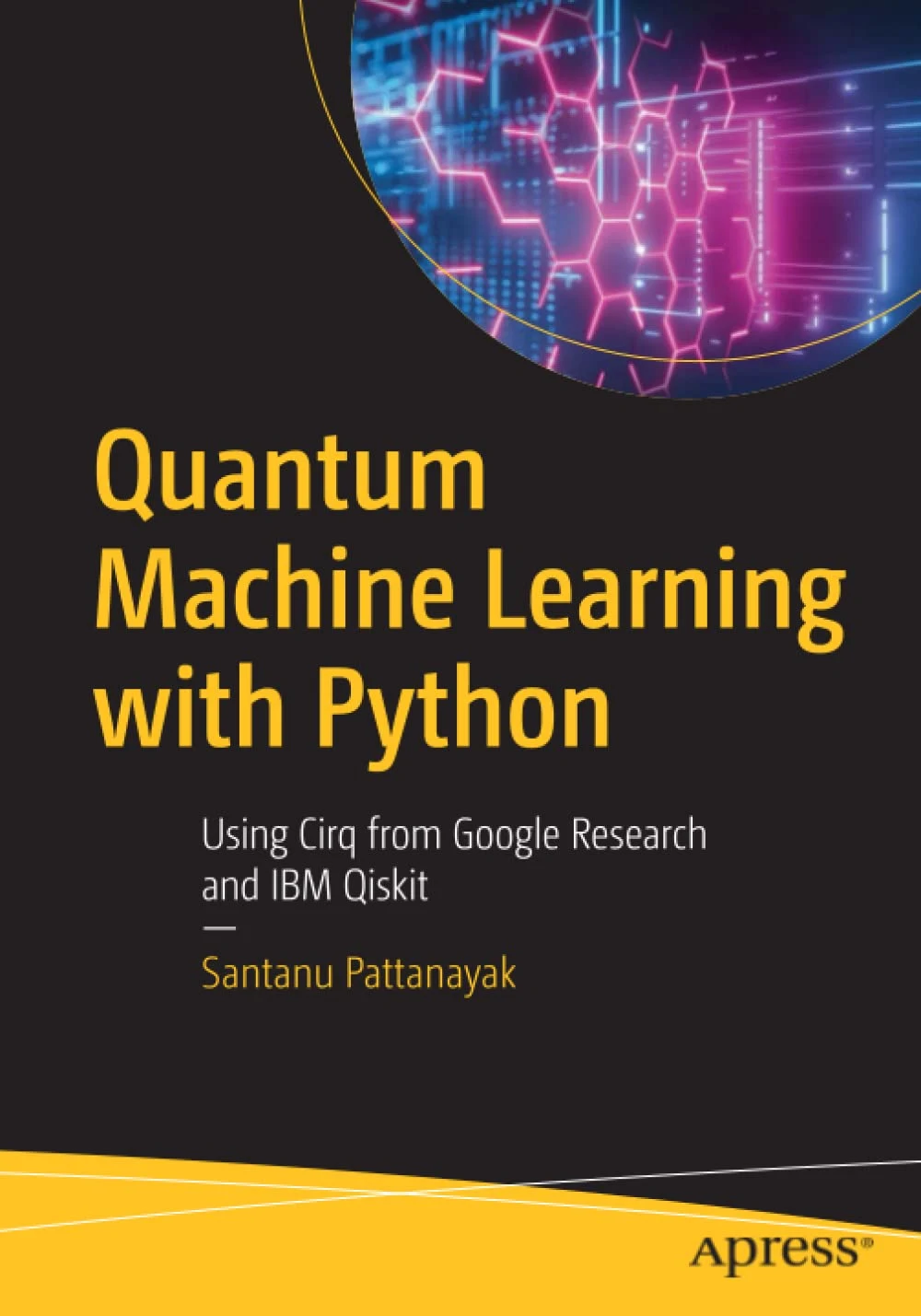
Quantum Machine Learning with Python: Using Cirq from Google Research and IBM Qiskit
Quickly scale up to Quantum computing and Quantum machine learning foundations and related mathematics and expose them to different use cases that can be solved through Quantum based algorithms.This book explains Quantum Computing, which leverages the Quantum mechanical properties sub-atomic particles.
Quantum Machine Learning (QML) emerges as a powerful crescendo, harmonizing the principles of quantum computing with the intricacies of machine learning. As we conclude our exploration into the realms of QML, it is evident that we stand on the precipice of a transformative era in computing and artificial intelligence.
The journey into the heart of QML reveals not only the technical marvels but also the collaborative spirit that drives its progress. Quantum physicists, computer scientists, and domain experts unite in a quest for knowledge, innovation, and solutions to some of humanity's most pressing challenges.
As we cast our eyes toward the future, Quantum Machine Learning stands as a testament to the relentless pursuit of understanding and the boundless potential of human ingenuity. The fusion of quantum computing and machine learning transcends the limits of classical paradigms, inviting us to explore a realm where computation is not just powerful but transformative.
In this age of rapid technological advancement, Quantum Machine Learning beckons us to imagine the unimaginable, to dream beyond the confines of what is known, and to embrace a future where the synergy of quantum and machine learning reshapes the very fabric of our technological reality. As the journey continues, so does the promise of unlocking new frontiers and reaching heights yet unexplored.
Related Articles:
- The Future of Blockchain Technology | Unlocking its Potential
- The Future of Machine Learning - Trends and Transformations
- Exploring the VR World - A Journey into Virtual Reality Technology
- Quantum Computing for Everyone - Demystified
- Demystifying Datafication | Transforming the World into Information
0 Comments